In the high school statistics chapter, we learned the concepts of regression function and regression analysis , and specifically studied linear regression , regression straight line equations and their applications . The following further summarizes the issues and applications related to extended linear regression.
In many practical problems, people can often only obtain part of the empirical data that reflects the characteristics of variables through observation or experiments. The problem requires us to start from these data to explore some hidden pattern or trend. If this pattern or trend does exist, and we can find a curve that approximately expresses this pattern or trend
,
Then, on the one hand, we can use this expression to summarize these data, and on the other hand, we can use it to predict other unknown values. The process of finding such a special curve type that fits specified data is called regression analysis, and the curve is called a regression curve.
The theory of regression analysis will not be covered until subsequent courses (such as probability and statistics courses). Here, we only introduce the simpler and widely used linear regression problem:
There are sets of empirical data
,
Make a scatter plot on the plane (see schematic diagram). If there is a roughly linear relationship between these data, the linear regression equation can be roughly determined :
,
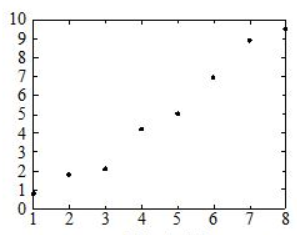
where is a specific coefficient related to the above empirical data:
,
.
Note: In high school , we introduced the linear correlation coefficient and its calculation The specific proof and derivation process of the above undetermined coefficients will be given later in the linear regression problem of learning the extreme value application of multivariate functions.